內容目錄
- Supervised Anomaly Detection
- Semi-supervised Anomaly Detection
- Unsupervised Anomaly Detection
- Anomaly Detection 技巧
Supervised Anomaly Detection
- 將所有的訓練資料以及測試資料集都進行標記
- 標準的機器學習都會使用這種方法(SVM, 神經網路)
Semi-supervised Anomaly Detection
- 標記少量的訓練資料,其中把所有正常的資料點都看成同一個class,但就只會有一組class存在而已,在測試資料中會混合anomalies,除了normal class之外的通通都辨識成異常值
- 所以通常叫做”One-class” classifier,SVM或者clustering可以辦到
Unsupervised Anomaly Detection
- 將normal以及anomalies都分在同一個dataset裡,並且不進行標注
- 如果特徵選得非常有代表性,讓normal以及anomalies可以精確分群的話是非常適合的方法,但困難的點是在normal裡既有的noise要如何跟anomalies進行分辨,以及為了精確的分群所以取的維度太高造成維度災難也是常常會發生的,維度要下降至何種程度常常需要不斷的實驗
Anomaly Detection 技巧
值觀想法
- 建構一組”normal”的行為資料:資料通常可以用統計的方法來形容,或者有某種樣態的分佈
- 使用這些”normal”的行為資料來進行偵測異常值:非常直觀的可以想像異常值應該會跟正常的資料型態長的天差地遠
方法
- Statistical-based
- Distance-based
- Model-based
統計方法
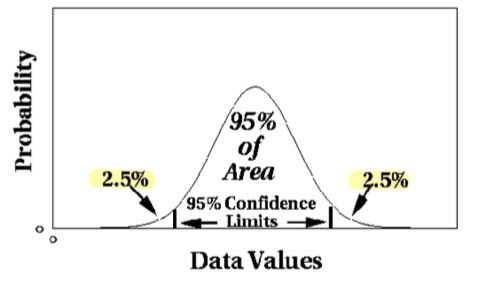
使用一組可調變參數化的model來描述某個資料集的分佈樣態,例如常見的就是normal distribution,同時在進行統計測試時常常會需要依據
- 資料分布
- 分佈的參數 (平均值,變異數等等)
- 預期中的異常值數量(confidence limit)